Diverging Paths: Exploring the Association Between Initial Math Pathways and College Students’ Subsequent Math Performance
Christine Mokher*
Florida State University
Shouping Hu
Florida State University
Abstract
Math pathways are an increasingly common policy reform where students choose a traditional math pathway (involving Algebra and/or Calculus) or an alternate math pathway more applicable to students' fields of study, namely Statistics or Liberal Arts Math with quantitative reasoning skills. We use data from all first-time-in-college students in the Florida College System to conduct an inverse-probability regression adjustment examining whether student's initial enrollment in different gateway math pathways influences subsequent math performance. We find the Liberal Arts pathways may increase the likelihood of students passing the first gateway course, but Algebra pathways tend to result in greater longer-term coursetaking success.
Introduction
Traditionally, college students have been required to complete a sequence of math courses focused on Algebra and Calculus even though the majority of non-STEM professions do not require these skills (Hacker, 2016; Handel, 2016; Rutschow & Diamond, 2015). These math courses tend to have low completion rates and may become a barrier to degree attainment for many students, particularly at community colleges (e.g., Bryk & Treisman, 2010; Huang, 2018). In response, reform initiatives at both the institution- and state-levels have promoted math pathways which provide students with an option of choosing a traditional math pathway (involving Algebra and/or Calculus) or an alternate math pathway centered on courses that are more applicable to their field of study, namely Statistics or Quantitative Reasoning (Burdman et al., 2018; Charles A. Dana Center, 2016). A national survey of developmental education practices found that approximately 41% of public two-year colleges offered multiple math pathways in 2016 (Rutschow & Mayer, 2018), and many of these efforts have continued to scale-up over time.
In addition to better aligning math courses with students' field of study, math pathways are intended to facilitate what Clifford Adelman (1999, 2006) calls "academic momentum." This concept suggests that the speed at which students start their college career will influence the trajectory of future academic success (Attewell et al., 2012). Recent literature, authored by researchers at the Community College Research Center, has identified three "early momentum indicators"-credit momentum, gateway momentum, and program momentum-that predict long-term college success, particularly higher completion rates (Jenkins & Bailey, 2017; Jenkins et al., 2017). Community college students are especially susceptible to momentum loss, as they are more likely to enroll part-time, work while they are enrolled, and "stop out" (i.e., take breaks in their enrollment), compared with their peers at four-year institutions (Monaghan & Attewell, 2015; Provasnik & Planty, 2008). For these reasons, students commonly find themselves delayed, sometimes for several semesters, by unsuccessful attempts to complete lengthy math course sequences. Now, with the availability of math pathways, those same individuals can access college-level math and major-specific courses much sooner, resulting in gateway and program momentum. Early studies suggest that math pathways may be an effective way to improve short-term outcomes like completion of college-level math credits (Jenkins et al., 2017; Rutschow, 2018; Rutschow et al., 2017; Schudde & Meiselman, 2019), as well as longer-term outcomes including transfer and degree attainment (Hoang et al., 2017; Norman, 2017).
In 2014, Florida implemented a statewide reform known as Senate Bill (SB) 1720 which allowed the majority of incoming college students to opt-out of placement testing and developmental education courses, and also required students to select meta-majors related to their intended field of study. Since then, Florida has been extensively exploring math pathways as a way to help students succeed (Florida Student Success Center, 2019). Students at all Florida College System (FCS) institutions are required to complete at least 6 semester hours of mathematics coursework at the equivalent of College Algebra or higher to fulfill the requirements for an associate in arts (AA) degree. Florida's common statewide course numbering system includes a sequence of eligible gateway courses in mathematics beginning with College Algebra (MAC X105), Liberal Arts Mathematics (MGF X106 or X107), or Statistics (STA X023)-herein referred to as initial math pathways. In this study, we explore whether there are effective math pathways that can be conducive to student success overall and for students who enter the math sequence at different academic levels. Specifically, we address the following research questions:
1. Does students' initial enrollment in different gateway math pathways (College Algebra, Liberal Arts Math, and Statistics) influence:
a) The likelihood of passing the first college-level math course?
b) The likelihood of completing the AA math degree requirements (2 or more college-level courses)?
2. Is there variation in the likelihood of success in each of the math pathways by students' lowest level of math enrollment (developmental, prerequisite, or gateway)?
This study seeks to make several important contributions to the extant research literature on math pathways. First, much of the prior research has focused on student success in the first college-level math course, but the question remains about whether students are adequately prepared to advance to the next level. In this study we examine how initial math pathway choices influence the likelihood that students will be able to successfully complete the associate's degree requirements in math within four years of college entry. Second, many prior studies have focused on examining the impacts of math pathways for all students, but it is important to see how outcomes may vary for students with different levels of academic preparation based on the level at which they enter the math sequence. Prior research has found differential effects of developmental education by level of college readiness (Boatman & Long, 2018), and these differences may continue into college-level coursework. Our study will shed light on the extent to which students' academic backgrounds may affect their chance of success in various math pathways, which should factor into decisions about how to assign students into different course options. These types of findings may inform practice among college advisors about the types of meta-majors and math pathways to recommend to students with differing levels of preparation.
In the following sections, we discuss three potential mechanisms through which math pathways can improve students' postsecondary success and then examine prior studies on the effectiveness of math pathways reforms. Then we describe the context in Florida in which math pathways were implemented to support larger reform efforts around developmental education and the adoption of meta-majors which group together programs of study that share common foundational skills. Next, we describe our methods which utilize student-level records from the population of incoming students at the 28 FCS institutions. We use inverse probability-weighted regression adjustment (IPWRA) to explore variation in success rates in completion of mathematics requirements over four years for similar students who select different math pathways. Finally, we present our results and the implications for policy and practice. If some math pathways do emerge to be more conducive to student postsecondary success overall and for students of different levels of preparation, then institutional leaders, practitioners, and state policy makers can use such findings to adjust implementation of math pathways to enhance student success and equity. These findings can also have significant implications for consideration in other contexts as guided pathways and math redesign has becoming increasingly popular reform options in other states (Bailey et al., 2015; Bickerstaff et al., 2018; Charles A. Dana Center, 2016).
Conceptual Framework
There are three potential mechanisms through which math pathways may improve students' postsecondary success. The first mechanism is academic momentum, which suggests that early improvements in success rates due to math pathways may lead students to develop self-efficacy and academic self-concept, resulting in greater commitment to degree completion (Attewell et al., 2012; Attewell & Monaghan, 2016). Belfield et al.'s (2019) study using data from community colleges in three states found that nine early indicators of credit momentum, gateway course momentum, and persistence momentum strongly predict longer-term completion rates. In addition, they found that early momentum metrics "strongly predict longer-term completion rates not only for students generally but also for Black and Hispanic students, suggesting that college efforts to close racial equity gaps in early momentum represent a crucial step toward closing gaps in credential completion" (p. 10).
The second potential mechanism underlying math pathways reform is structural changes to math course placement. College placement tests tend to disproportionately underplace students into developmental education courses when many of these students likely could have passed college-level courses (Leeds & Mokher, 2020; Scott-Clayton et al., 2014). Further, standardized tests tend to have less predictive strength for students of color who are more likely to underperform relative to their true abilities (e.g., Bowen et al., 2018; Hoffman & Lowitzki, 2005). Florida's larger reform efforts remove the requirement for placement testing, and the availability of new math pathways may allow students to progress more quickly into college-level courses.
The third potential mechanism of math pathways is the removal of potentially unnecessary Algebra requirements, which could address potential disadvantages in prior academic preparation among underrepresented subgroups of students. Academic preparation is developed in K-12 schools, and Black and Hispanic students are more likely to come from underperforming and underfunded schools. The challenges that these students face from underpreparation may be further exacerbated in community colleges that tend to provide insufficient support such as advising and tutoring, as well as misalignment between developmental and college-level courses (Melguizo et al., 2008). According to the Florida Student Success Center (2019), the availability of alternate math pathways has the potential for reducing the breadth of topics and/or skills in individual and required courses over traditional Algebra-based pathways, which suggests that the reform should increase the likelihood of successful completion for students with weaker prior academic preparation. There is also some evidence that students with math anxiety tend to avoid higher-level math courses and STEM-based courses (Purnell & Burdman, 2021), or may delay taking math courses until the end of their academic program (Hu et al., 2016). If these students have previously struggled with algebra courses in high school, providing alternatives to College Algebra may provide them with greater confidence which may ultimately result in an increased likelihood of math success.
Literature Review
The discussion around math pathways is commonly connected to a larger conversation about the need for developmental education reform. Math is the most commonly needed remedial class, nationally (Bahr, 2007) and in the state of Florida (Underhill, 2013). Unfortunately, only 20% of students referred to developmental math actually complete a college-level math course within three years' time (Bailey et al., 2010; Rutschow & Diamond, 2015). For this reason, critics of remedial math curricula label it as a gatekeeper, rather than a gateway, to higher education (Bryk & Treisman, 2010; Huang, 2018). These negative outcomes are often attributed to a "pipeline effect," whereby students "leak" out of remedial pathways between classes, often before getting to college-level work (Charles A. Dana Center, 2016; Hern, 2010).
Meta-majors, and their associated math pathways, have been offered up as one solution to this problem of large-scale attrition. The most notable math pathways models in use by these institutions are the Dana Center Math Pathways (DCMP), the American Association of Community Colleges (AACC) Pathways Project, and the Carnegie Math Pathways. While none of these models remove the option of developmental education entirely, they tend to reduce the length of time students spend in remedial course sequences by one or two semesters. Math pathways also attend to the rising concern that algebraic competencies are not well-linked to the needs and expectations of most professions by allowing students in non-STEM meta-majors to enroll in Statistics or Quantitative Reasoning courses (Hacker, 2016; Handel, 2016; Rutschow & Diamond, 2015). Rather than channeling most students through College Algebra, as was previously done, math pathways route those in the social sciences and business professions down a Statistics pathway and those in the humanities and liberal arts down a Quantitative Reasoning pathway (Rutschow, 2018).
Early findings indicate that math pathways may be an effective way to move students through the higher education pipeline. For instance, the Carnegie Foundation for the Advancement of Teaching boasts that their model continues "to deliver three to four times the success rate of traditional pathways in half the time," even as participation levels have increased dramatically over the past six years (Hoang et al., 2017, p. 14). More specifically, participation in their pathways program is associated with improved outcomes related to accumulation of college credit, transfer, and degree attainment (Hoang et al., 2017; Norman, 2017). These findings have continued to hold as the model was scaled up to new institutions, and results were consistent across gender and racial student subgroups (Huang & Yamada, 2017; Yamada et al., 2018).
An evaluation of the DCMP model revealed that participation in math pathways increases the likelihood that students enroll in and complete more college-level math credits than their peers in the "standard" pathway (Rutschow, 2018; Rutschow et al., 2017). Another study found that students in DCMP developmental math courses were 5 to 9 percentage points more likely to complete a college-level math course within one semester than their peers in traditional developmental math courses (Schudde & Meiselman, 2019). Individual colleges participating in the AACC Pathways Project report similar findings. At Lakeland Community College in Ohio, pathways reform increased the rate of first-time students taking and passing college-level math in their first year from 12% to 21%. At another Ohio college, North Central State College, more than 75% of students took Statistics instead of College Algebra, with pass rates for college-level math during a student's first year at 34%, instead of 20% prior to implementation (Jenkins et al., 2017).
How math pathways are designed has some bearing on student success. For instance, research points to the importance of timing and continuity of math enrollments for college outcomes. Some suggest that the best time to take a math course is during one's first semester of college; those who do tend to experience higher rates of degree attainment and transfer (Adelman, 2005). And, once students get started in a math pathway, it is important that they keep going in order to maintain momentum. The Charles A. Dana Center (2015) has identified back-to-back enrollment of math courses as a promising practice. When students move directly from one math course to the next, especially as a cohort, rates of gateway course completion increase. Math pathways encourage back-to-back enrollment by presenting students with an educational roadmap that details a clear sequence of courses spanning several semesters. Math pathways that utilize defaults go one step further by enrolling students automatically in the next math course; students who want to take a break must make additional efforts to opt out (Bailey et al., 2015). It is also worth noting that there may be some pathways that are more economical and efficient than others when it comes to helping students reach their academic goals (Finster & Feldman, 2021).
Study Context
The FCS is one of the two major public postsecondary systems in the state of Florida. The FCS is composed of 28 public community and state colleges with enrollment estimated at nearly 800,000 students at 70 campuses across Florida. These colleges offer a host of programs, including bachelor's degrees, associate's in arts and associate's in science degrees, college credit certificates, vocational credits, college and vocational preparatory programs, and life-long learning courses (Florida College System, 2018). The FCS serves as the primary point of access to higher education credentials in Florida. Sixty-five percent of Florida's high school graduates who continue to college do so by enrolling in an FCS institution. That percentage increases to 82% for non-White freshman and sophomore students from historically underrepresented populations (Florida College System, 2018). The other public postsecondary system is the Florida State University System (SUS), which includes the state's 12 universities providing undergraduate, graduate and professional education, research, and public service. Upon completion of an AA degree from a Florida two-year public institution, Florida statute guarantees the opportunity to attend a four-year public institution within the SUS to earn a bachelor's degree.
Figure 1 illustrates the math courses taken under traditional and reformed math sequences in Florida for students seeking an AA degree. Under the traditional sequence, students are required to enroll in a developmental math course if they score below college-ready on a placement test. Next, most students are required to enroll in a prerequisite math course (most commonly Intermediate Algebra, or MAT 1033) which counts for elective credit but does not fulfill the degree requirements in math. Upon completion of these non-degree courses (or with approved scores on exams such as ACT or SAT) students enroll in College Algebra (MAC 1105) as the first course to fulfill the AA degree requirements in math, and then take a second more advanced course such as Precalculus.
Figure 1. Comparison of Courses under the Traditional Math Sequence and Reformed Math Sequence
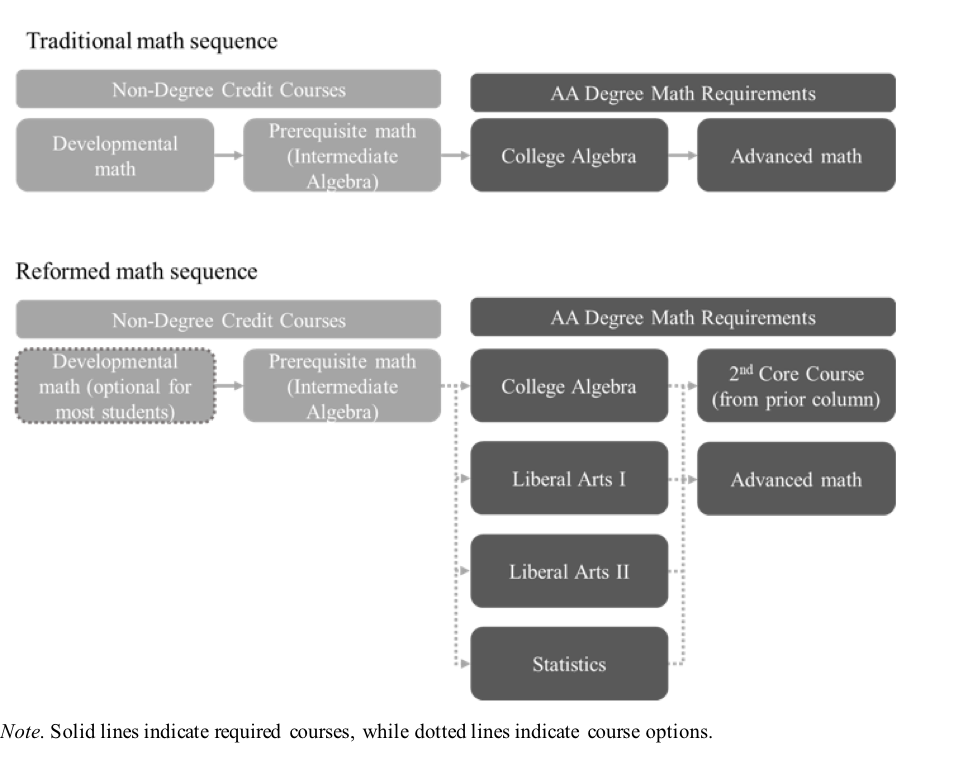
Beginning in Fall 2014, Florida SB 1720 mandated changes in developmental education (DE) in the FCS, specifically in terms of how DE is taught and for whom it is required in the FCS (Park et al., 2016). Specifically, exempt students, such as students who are active duty in the armed forces or entered high school in the 2003-04 academic year or after and earned a standard diploma from a public high school in Florida, now have the option to bypass DE and enroll directly in college-level English and math coursework. The legislation also required all colleges to develop meta-majors, or a collection of programs of study or academic discipline groupings that share common foundational skills (e.g., humanities, social and behavioral sciences, business, or health sciences).
Under the reformed math sequence, most students can opt out of developmental math, but are still required to take prerequisite math course. They are also still required to complete at least 6 semester hours of mathematics coursework at the equivalent of College Algebra or higher to fulfill the requirements for an AA degree. However, additional options have been added regarding the types of courses that can be used to fulfill the math degree requirements. Florida's common statewide course numbering system includes a sequence of eligible core courses in mathematics beginning with College Algebra, Liberal Arts Mathematics (MGF 1106 or MGF 1107),1 or Statistical Methods (STA 2023). We refer to the first course selected from this list as the student's "initial gateway math pathway." While colleges are not required to offer all three pathways, many institutions began expanding the availability of the three pathways in response to the meta-majors mandate so that math courses could be better aligned with students' program of study. After completing the first core math course, students have the option to complete either a second core course or a more advanced course to complete the AA degree requirements. While students are advised to take a sequence of courses in the same pathway (e.g., from Liberal Arts I to Liberal Arts II), they have the option to take a second course from a different pathway.
Florida is engaging in a multi-pronged effort to implement guided pathways at scale, incorporating strategies such as academic degree mapping, re-designing onboarding and advising services, and articulation for seamless transfer. Math redesign and content alignment are among the primary considerations. In 2018, the FCS partnered with Jobs for the Future, Helios Education Foundation, and the Florida College System Foundation to establish the Florida Student Success Center (FSSC), which further promoted the development of math pathways. FSSC's first charge was to develop a coherent statewide strategy for math pathways redesign and content alignment, and to share best practices on math pathways across institutions. This collaborative P-20 effort seeks to further improve access to and quality of math pathways throughout the state's education system. Since then, Florida has been extensively exploring both guided pathways and math redesign as ways to help students succeed in the FCS (Florida Student Success Center, 2019).
Methods
We use data from Florida's P-20 Educational Data Warehouse which includes all 28 public institutions in the FCS. Our sample consists of FCS students from the 2014 first-time-in-college (FTIC) cohort who ever enrolled in any gateway math course between Fall 2014/15 and Spring 2017/18. The dependent variables include two dichotomous outcomes for (a) whether the student completed the first gateway math course, and (b) whether the student completed the AA math degree requirements (2 or more college-level courses) within four years of college entry, where 1=completed and 0=did not complete. Students who drop out of college are coded as 0 for not completing the course rather than missing. Data are not currently available on whether students attained a degree, but these outcomes reflect students' progress toward degree completion and allow us to examine both short and longer-term outcomes.
Independent variables include student race/ethnicity, gender, free- or reduced-price lunch status in high school, language minority status, verified disability status, age, years since high school graduation, type of high school diploma (standard, GED, or other), and number of credits enrolled during the term of the initial gateway course. The models also include controls for high school coursetaking track where "at-risk" indicates students who failed one or more high school math courses, "basic" indicates students who passed all courses but did not complete Algebra II, "standard" indicates students who completed math courses up to Algebra II, and "advanced" indicates students who completed Algebra II and at least one more advanced math class.
Table 1 provides descriptive statistics for the independent variables among the analytic sample of students who ever enrolled in any gateway math course compared to the population of all students in the 2014 FTIC cohort. The dummy variable adjustment method is used to handle missing data among the independent variables where the missing value is set to a constant of 0 and additional dummy variables are added to the model to indicate whether there is missing data for the actual value (Cohen & Cohen, 1983). The extent of missing data is relatively minor at less than 3% for most variables, with the exception of the high school coursetaking variables which are missing for 18% of the analytic sample. Students may be missing high school records if they attended a private school, out-of-state school, or home school; as well as older students who attended a public high school prior to the development of Florida's state longitudinal data system in the 1990s.
Table 1. Descriptive Statistics for the Analytic Sample of Students Who Ever Enrolled in Any Gateway Math Course Compared to the Population of All Students in the 2014 FTIC Cohort
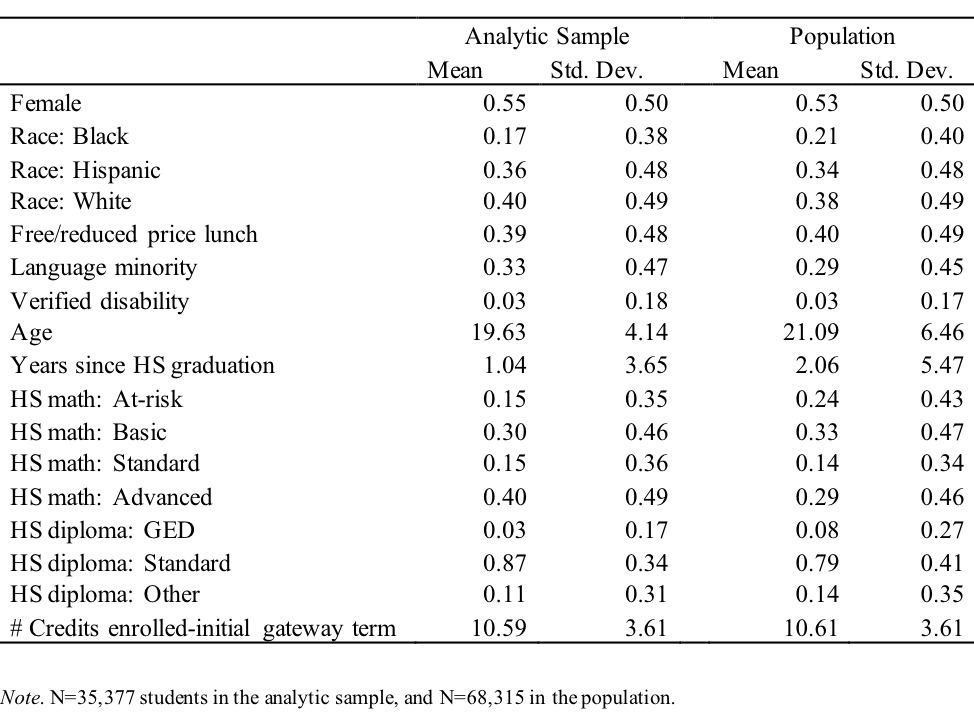
We use propensity score reweighting for exploratory comparative purposes to examine student success by math pathways. Matching methods reduce the selection bias that is associated with nonrandom assignment into a given math pathway by utilizing covariates to make treatment and outcomes independent after conditioning on those covariates. One limitation of this approach is that there still may be some remaining differences on unobservable characteristics such as student motivation; however, when randomized trials are infeasible matching can be used to balance samples on observable characteristics to allow for more rigorous inferences compared to other statistical methods (Reyonds & DesJardins, 2010). This type of approach has been used in other studies examining the impact of math pathways on postsecondary outcomes when random assignment was not possible (e.g., Huang & Yamada, 2017; Schudde & Keisler, 2019; Yamada et al., 2018).
In order to account for differences in the types of students who may be enrolled in each math pathway, we use IPWRA which can account for multi-valued treatments where each subject could receive one of multiple options for treatments. For this study, the traditional pathway, College Algebra, serves as the "untreated" group (t=0) and there are separate "treatment" groups for the Liberal Arts pathway (t=1) and the Statistics pathway (t=2). We want to know how effective each of these alternate pathways are relative to the traditional pathway, and then compare the effectiveness of the Liberal Arts pathway with that of the Statistics pathway. While ideally we would also like to estimate the models separately for each racial/ethnic group to explore whether there are effective math pathways that can be conducive to success for students of different backgrounds, many of the sample sizes are too small to generate adequate matches when further disaggregated by student demographic characteristics.
IPWRA is a doubly robust approach in which a multinomial logistic regression is estimated in the first stage for the probability of enrollment in each of the alternate math pathways relative to the College Algebra pathway (the traditional pathway). We estimate the following model for each individual i:

where P is the probability of selecting into individual treatment (t) among (T) available treatments. β0t is an intercept and X is a matrix of coefficients for covariates including the student background characteristics, high school academic preparation, and postsecondary variables shown in Table 2. Rather than matching individual observations to others, the propensity scores are used to reweight each observation (Reynolds & DesJardins, 2010). Weights (W) are defined as the inverse of the generalized propensity score as follows:

This creates a pseudo-population where cases are weighted so that covariates have a similar distribution across each of the treatment options (Leite et al., 2019).
Table 2. Covariate Balance with Standardized Mean Difference for Each Initial Math Pathway Relative to the College Algebra Pathway for the Unmatched and Matched Full Samples
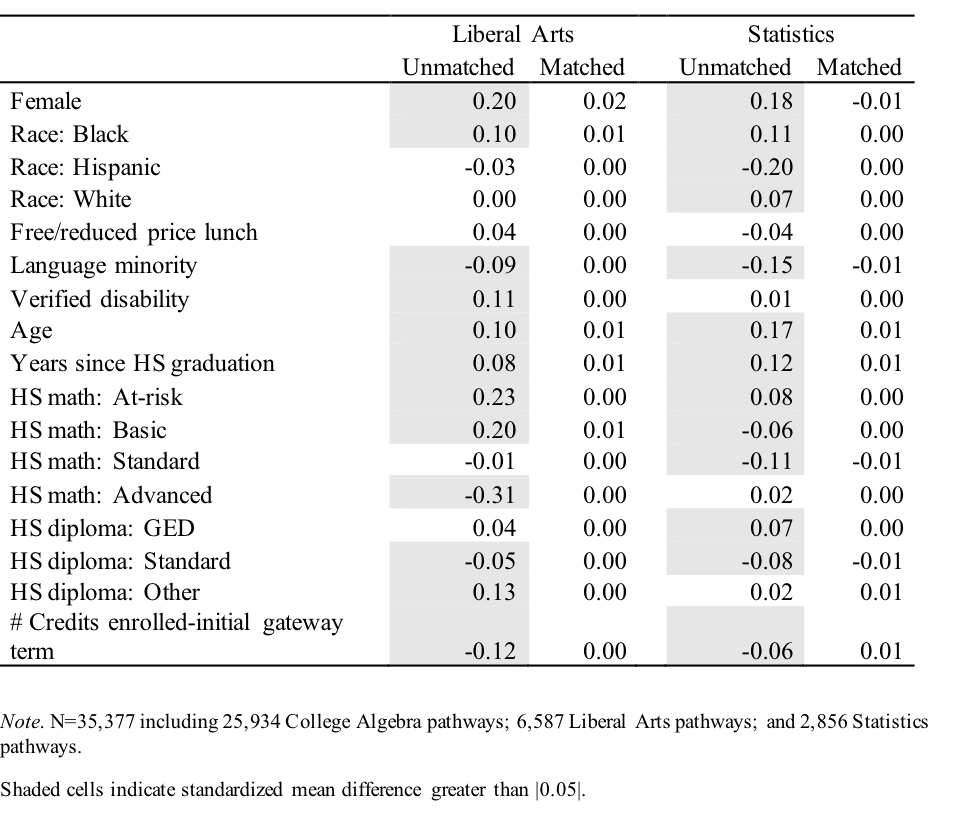
In the second stage, regression adjustment is used to predict the likelihood of the outcomes to explore the extent to which each math pathway is associated with the outcomes of interest. We estimate models for the dichotomous outcomes of completing various math courses using a linear probability model as follows:
yi = γ0 + γt + ΓX + εi, with inverse probability weights (WiT) based on P(t1).
yi is the outcome of interest (completed course=1; did not complete course=0), γ0 represents the intercept, γt is the effect of participation in treatment t, X is the matrix of coefficients for covariates representing student characteristics, and εi is the residual term. Outcomes among students with a likely treatment receive a weight close to one, whereas outcomes of individuals with an unlikely treatment receive a weight greater than one. Standard errors are adjusted to reflect the uncertainty associated with the predicted treatment probabilities. For ease of interpretation, we also present the results as predicted probabilities of passing each course type by initial math pathway.
Diagnostic checks are used to ensure the plausibility of the overlap assumption and evaluate covariance balance after weighting. The overlap assumption states that each individual has a positive probability of receiving treatment, which ensures that the predicted inverse-probability weights do not get too big. We diagnose this assumption visually with a histogram that plots the estimated densities of the probability of taking each math pathway. As shown in Figure 2, we find similar distributions for the estimated densities of the probabilities of each initial math pathway which suggests that the overlap assumption is not at-risk of being violated.
Figure 2. Estimated Densities of the Probability of Each Initial Math Pathway, Overall and by Lowest Level of Math
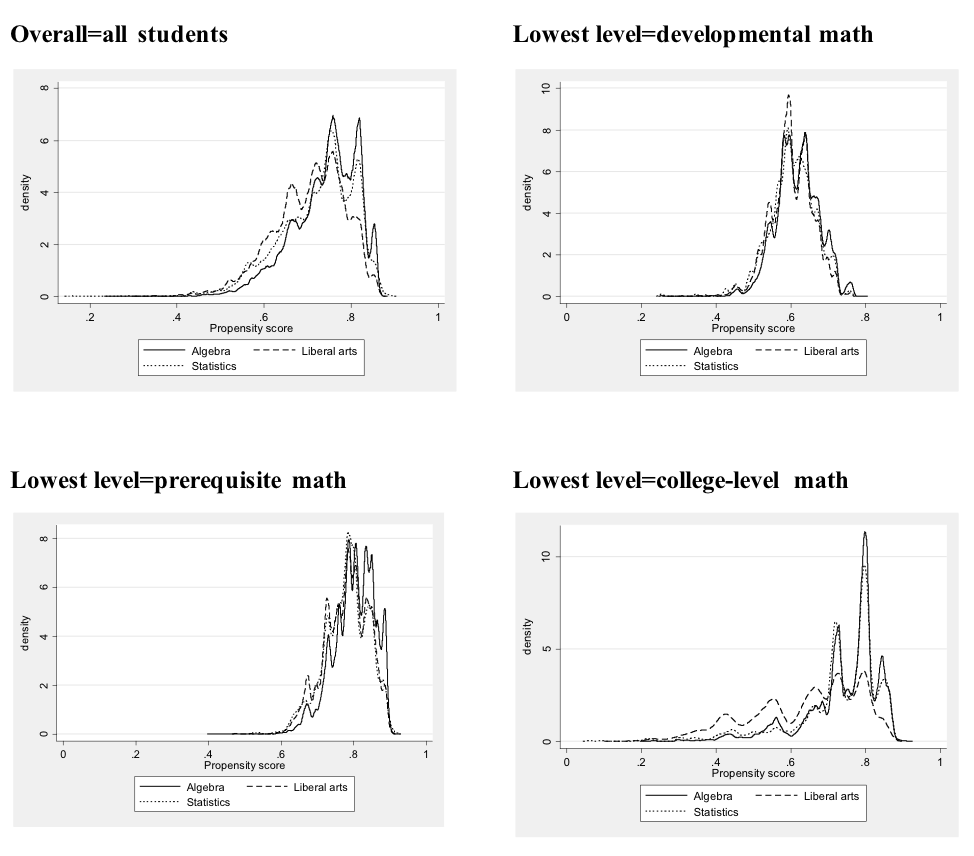
Next, we calculate the standardized mean difference for each covariate among students in the traditional math pathway relative to students in each of the alternate math pathways in order to assess covariance balance after weighting. The What Works Clearinghouse (2020) specifies that differences less than 0.05 standard deviations demonstrate acceptable covariate balance, or differences of less than 0.25 standard deviations with additional covariate adjustment. In our data, the standardized mean differences among the independent variables for each of the alternate math pathways relative to the traditional pathway range from 0.00 to 0.23 prior to matching (Table 2). However, after matching, all standardized mean differences are less than 0.05 standard deviation units in the full sample. Similar results emerge when the baseline equivalence is tested separately for each of the subgroups based on the lowest level of math. The one exception is a standardized mean difference of -.06 for number of credits enrolled in the initial gateway term for the Statistics pathway relative to the College Algebra pathway. All of our models include a full set of covariates (X) to account for any small imbalances between math pathway groups after the inverse probability weighting.
Findings
We begin by presenting descriptive statistics on math pathways, followed by the regression-adjusted predicted probabilities of student outcomes by pathway. Despite the availability of alternate math pathways, nearly three-quarters of students (73.3%) enrolled in the traditional College Algebra pathway (Table 3). Only 18.6% of students enrolled in the Liberal Arts pathway and 8.1% of students enrolled in the Statistics pathway for the initial gateway course. There was some variation among students who entered the math sequence at different levels, as students who started in developmental math were the least likely to enroll in the College Algebra pathway (61.2%, relative to 79.7% for students who started in prerequisite math and 72.2% for students who started in gateway math) and most likely to enroll in the Liberal Arts pathway (30.6%, relative to 13.3% for students who started in prerequisite math and 18.0% for students who started in gateway math). Statistics was the least common pathway, comprising less than 10% of initial gateway math enrollments across all three groups of students by initial math level.
Table 3. Number (and Percent) of Students Who Took Their Initial Gateway Math Course in Each Pathway
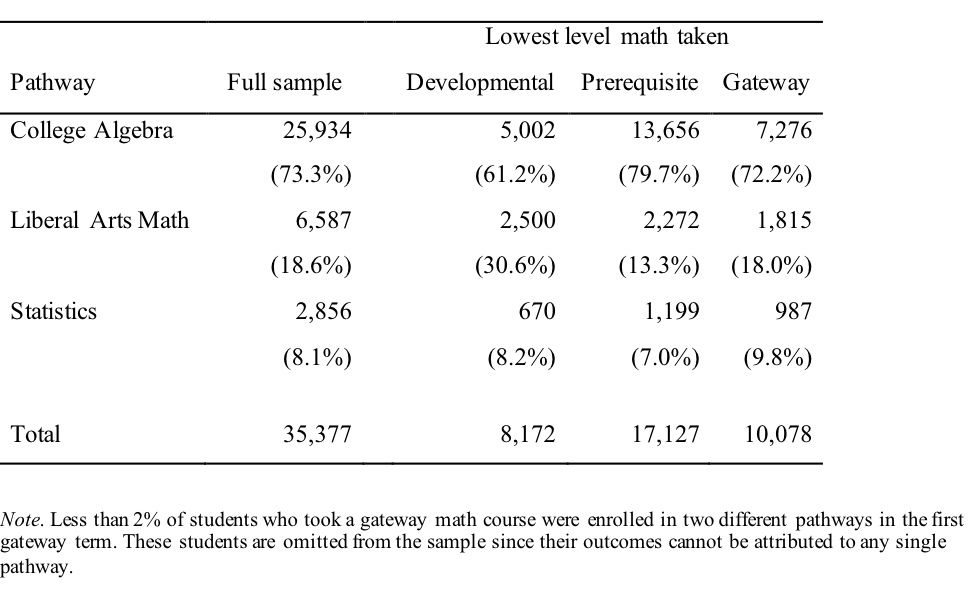
The predicted probabilities from the IPWRA models indicate that the likelihood of completing the initial gateway math course ranged from 70% for the Statistics pathway to 78% for the Liberal Arts pathway in the full sample (Figure 3). Across all models, the likelihood of completing the first gateway course was higher for students on the Liberal Arts pathway relative to the College Algebra pathway (Table 4). The magnitude of the difference was greatest among students whose lowest math was developmental or prerequisite, where the likelihood of gateway course completion was about 5 percentage points higher for the Liberal Arts pathway relative to the traditional College Algebra pathway. The results also indicated a negative effect of the Statistics pathway relative to the College Algebra pathway in most models. For the full sample, the likelihood of completing the initial gateway math course was 76% for the College Algebra pathway versus 70% for the Statistics pathway, a difference of 6 percentage points. The effect was even greater among the developmental math subgroup, where the likelihood of gateway math completion was 71% for the College Algebra pathway relative to 61% for the Statistics pathway, a difference of 10 percentage points. However, there was no statistically significant difference for the subgroup of students whose lowest math was a gateway course.
Figure 3. Predicted Probabilities of Completion of Subsequent Math by Math Pathway
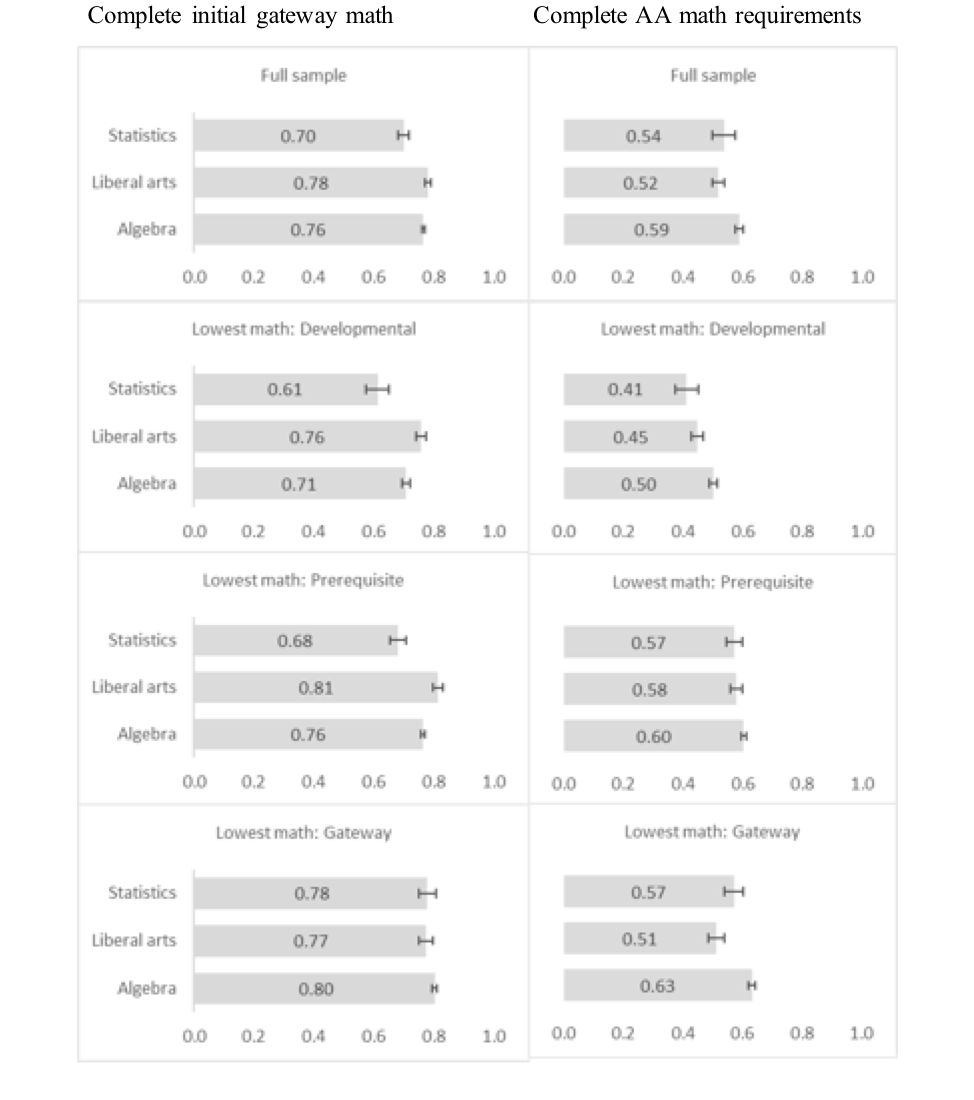
Table 4. Relationship Between Initial Math Pathway and Outcomes: Average Marginal Effects and Control Group (College Algebra Pathway) Means
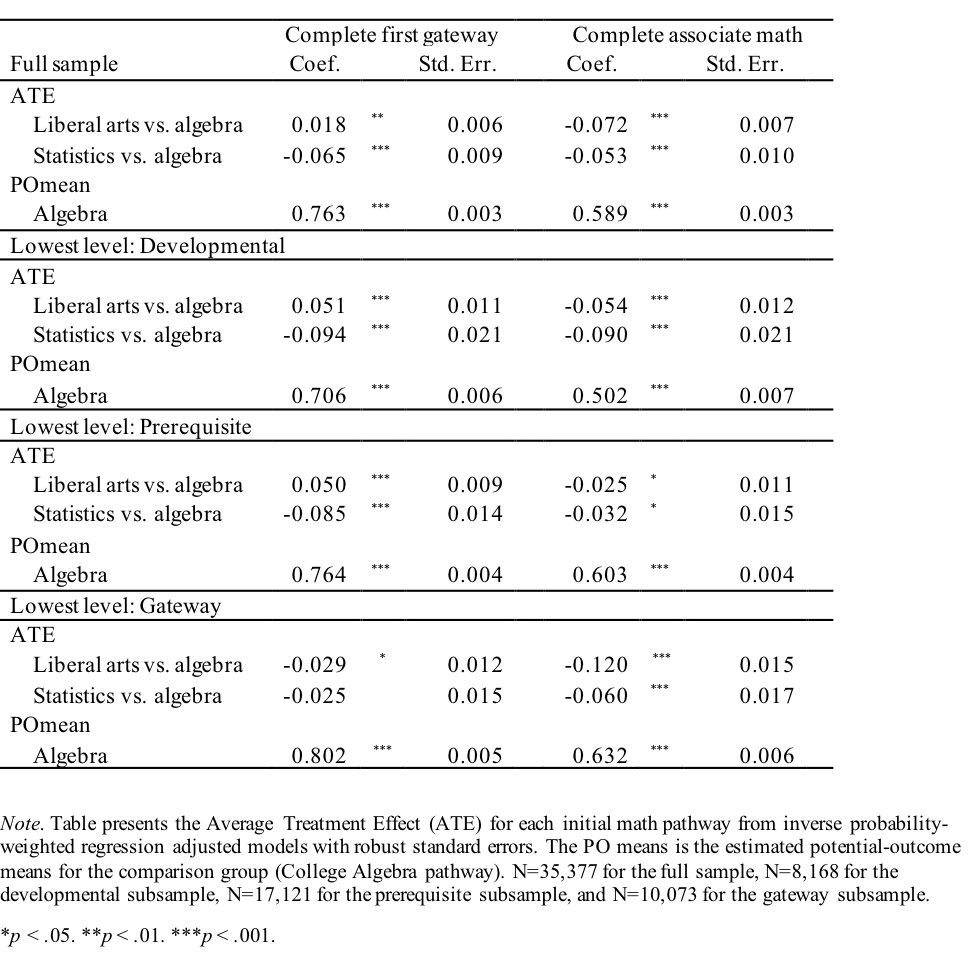
A slightly different set of results emerged for the outcome of completion of the AA math degree requirements. Among the full sample, the likelihood of a successful outcome ranged from 52% for the Liberal Arts pathway to 59% for the College Algebra pathway (Figure 3). Across all models, the likelihood of completing the AA math degree requirements was lower for students on both the Liberal Arts and Statistics pathways relative to the College Algebra pathway (Table 4). The greatest differences by pathway were for students whose lowest math was a gateway course, with a 63% likelihood of completing the AA requirements for the College Algebra pathway compared to 51% in the Statistics pathway, a difference of 12 percentage points.
To further examine coursetaking patterns after the first gateway course, we looked at the percent of students who took different types of subsequent gateway or advanced math courses (Table 5). About one-third of students did not attempt a second math course, ranging from 27.6% in College Algebra to 36.7% in Liberal Arts math. Among the students who did take a second math course, many did not continue on the same math pathway as their initial gateway course. For example, College Algebra has traditionally been designed to lead into Precalculus or Calculus courses, yet only 36.9% of students in our sample who started on the College Algebra pathway took this route. Instead, students who initially took the College Algebra pathway were more likely to enroll in Statistics (47.7%) and some also enrolled in a first or second Liberal Arts math course (13.1% and 2.0%, respectively) or another advanced math course (8.2%). We might also expect that most students who initially took a Liberal Arts math course would enroll in a second Liberal Arts college-level course-yet only about one-third of the Liberal Arts students in our sample (34.0%) did so. Students on the Statistics pathway also had varied subsequent math coursetaking patterns, as most students in this subgroup took College Algebra (27.3%), a first Liberal Arts math course (26.7%), and/or a Precalculus or Calculus course (23.2%).
Table 5. Percent of Students Who Took Subsequent Gateway or Advanced Math Courses, by First Gateway Math Pathway
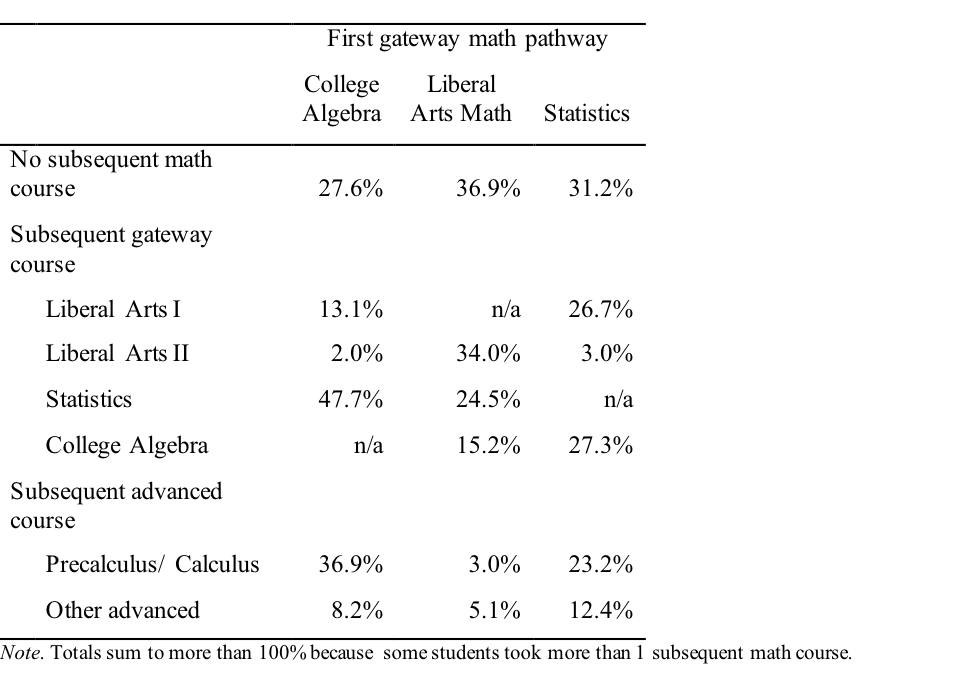
Discussion
This study sought to examine differences in the likelihood of completing initial and subsequent math course requirements for similar students on different math pathways among the population of FTIC students in Florida state colleges. Overall, our results indicate that the Liberal Arts pathway may increase the likelihood of students passing the first gateway math course (relative to the traditional College Algebra pathway), but these gains may not persist to longer-term outcomes. Instead, students on the College Algebra pathway are more likely to complete AA math degree requirements than those on either the Liberal Arts or Statistics pathways. These findings demonstrate the importance of looking beyond first-year coursetaking outcomes to consider the implications of math pathways for longer-term student success.
It is also important to consider the specific policy context in Florida when interpreting these results. The timing of the scale-up of math pathways occurred simultaneously with larger reform efforts that made developmental education optional for the majority of incoming students and required colleges to organize their programs of study around meta-majors with similar foundational skills. It is worth noting that due to the freedom in course selection, students who enroll in the same gateway math courses (either Liberal Arts, College Algebra, or introductory Statistics) have varied pre-gateway starting points due to their different choices of enrolling in or skipping developmental math. While the majority of students selected the College Algebra pathway regardless of their initial math enrollment, students who started in developmental education courses were more likely than those with other initial enrollment levels to choose one of the alternate math pathways. Yet their outcomes were similar to those in the full sample, as students who started in developmental education and enrolled in the College Algebra pathway were less likely to pass the first college-level course but more likely to pass a subsequent math course relative to similar peers in other math pathways.
We posit that one potential explanation for the difference in effects between the first and second math course outcomes is that many students do not continue on the same pathway for subsequent math courses, and the content of the College Algebra pathway may be focused more on foundational skills that are applicable to different types of subsequent courses. Another possibility is that taking a higher-level initial math course provides students the flexibility to take another similar or slightly less rigorous course while still meeting the requirements for degree completion. Additionally, a recent study of faculty perspectives regarding the Quantway alternative pathway for non-STEM majors found that while there tended to be positive aspects of the program like improved student motivation, some faculty questioned whether these courses had an appropriate level of academic rigor (Cafarella, 2021). If courses on alternate pathways tend to be less rigorous than traditional math sequences, then students in these pathways may struggle more when progressing to subsequent math courses. This aligns with prior research by Bahr et al. (2017) which found that students who entered college STEM coursework from less rigorous courses tended to be less likely to advance to higher-level courses relative to students who entered their coursework at a slightly more rigorous level. Future research is needed to explore how success rates differ depending on coursetaking patterns after the initial gateway course. Researchers should also consider whether there are any differences in outcomes over time due to confounding factors such as the shift to online or hybrid courses at both the high school and college-levels following the COVID-19 pandemic.
The findings from this study may inform important decisions for both policy and practice. Current models of postsecondary education are rife with choices for students. Students choose everything from their college and degree program to their course load (full-time versus part-time), program electives, and preferred learning environment (e.g., online versus face-to-face). Prior research has shown that college students often make uninformed decisions about course selection, and that the pathways chosen by community college students are often misaligned with students' likelihood of success in terms of the predicted probabilities of completing programs and attaining high earnings (Holzer & Xu, 2019). Scott-Clayton (2011) contends that students do not often make wise choices because they are not cognizant of their needs, cannot adequately sort through and process all of the information available to them, or defer important decisions until deadlines have passed. Community colleges structures are particularly complex due to the multiple missions (e.g., the collegiate, vocational, remedial, contract, and community service missions) such institutions pursue (Cohen et al., 2013). Guided pathways intend to address many of these problems by simplifying academic programs, limiting choice, and introducing default course sequences. Yet Florida's math pathways model provided students with more choices which may have introduced additional complexity. Given that the results of our study show that many students do not continue on the same math pathway, it seems that improvements may be needed about how information is disseminated about math pathways and the type of guidance that students receive from advisors about their options.
Another important consideration for policy and practice is the implications of the initial math pathway selection for success in future courses and beyond. One of the recommendations from the Florida Student Success Center (2019) is to create common math pathways aligned to meta-majors, which would include limiting College Algebra to students in STEM and certain business majors only. Yet our study shows that there are relatively few differences in the likelihood of passing the first college-level math course for higher performing students who start at the gateway course level, and most students (regardless of the initial level of math enrollment) tend to have a higher likelihood of success in subsequent math courses if they are on the College Algebra pathway. There may still be some unobserved characteristics, such as student motivation, that may contribute to differences among students in different math pathways so it would not be reasonable to assume that all students should take College Algebra. However, it also seems that rather than imposing a single math pathway upon all students in a meta-major, it may be preferable to provide personalized advising that takes into account factors such as prior math coursetaking in high school and students' level of certainty of their intended majors. College Algebra may be needed if students want to switch majors or transfer to a four-year university with different degree requirements. This has also been an issue in other contexts such as California, where some community colleges have been hesitant to expand Statistics pathways due to concerns about whether these courses would be transferable to four-year universities for some majors (Mejia et al., 2019). Ensuring applicability and transferability of courses has been identified as a critical dimension of effectively scaling math pathways among statewide reform efforts (Bickerstaff et al., 2018).
There is also a need for future research to examine the moderating roles of student characteristics such as gender and race/ethnicity in the relationship between math pathways and success. Prior research has shown disparities in STEM coursetaking by student characteristics such as race and gender (Bahr et al., 2017; Wang, 2016), and changes to math pathways may have the potential to reduce gaps in attainment. Students from underrepresented racial and ethnic groups may face even greater challenges in developmental and gateway math courses due to issues of discrimination and racism, structural inequalities, as well as a failure of institutions to support their unique social and cultural values (e.g., Harper et al., 2009; Mueller & Broido, 2012). There is also evidence of inequality in math tracking, with Black and Latinx students having the greatest likelihood of falling into a "math trap" in college where they take courses that repeat content from high school, even after controlling for prior grades (Ngo & Velasquez, 2020). Given that prior research has found students of color have a higher likelihood of underperforming in mathematics and being placed into lower-level math course tracks compared to their more advantaged counterparts, they also stand to benefit more if math pathway reforms are effective. There is already some promising evidence from the Carnegie Math Pathways that Black females had the largest gains in mathematics achievements among all student subgroups (Huang & Yamada, 2017; Yamada et al., 2018). The DCMP has also demonstrated significant improvements in the engagement of students of color, and their learning outcomes and pass rates (Charles A. Dana Center, 2016). If some math pathways do emerge to be more conducive to postsecondary success for students of different backgrounds, then institutional leaders, practitioners, and state policy makers can use such findings to adjust implementation of math pathways to enhance student success and equity. Such an effort is critical for student postsecondary success and equity in the state's open access institutions. It can also have significant implications beyond Florida as guided pathways and math redesign has gained steam in many states (Bailey et al., 2015; Charles A. Dana Center, 2016).
Footnote
1The two courses for Liberal Arts Mathematics largely cover different topic areas. MGF 1106 includes systematic counting, probability, statistics, history of mathematics, geometry, sets, and logic. MGF 1107 includes financial mathematics, linear and exponential growth, numbers and number systems, history of mathematics, elementary number theory, voting techniques, and graph theory. Some colleges require or recommend MGF 1106 to be taken before MGF 1107, while other colleges allow for either course to be taken first.
References
Adelman, C. (1999). Answers in the toolbox: Academic intensity, attendance patterns, and bachelor's degree attainment . U.S. Department of Education, Office of Educational Research and Improvement. https://files.eric.ed.gov/fulltext/ED431363.pdf
Adelman, C. (2006). The toolbox revisited: Paths to degree completion from high school through college. U.S. Department of Education, Office of Vocational and Adult Education. https://www2.ed.gov/rschstat/research/pubs/toolboxrevisit/toolbox.pdf
Adelman, C. (2005). Moving into town-and moving on: The community college in the lives of traditional-age students. U.S. Department of Education, Office of Vocational and Adult Education. https://files.eric.ed.gov/fulltext/ED496111.pdf
Attewell, P., Heil, S., & Reisel, L. (2012). What is academic momentum? And does it matter? Educational Evaluation and Policy Analysis, 34(1), 27-44. https://doi.org/10.3102%2F0162373711421958
Attewell, P., & Monaghan, D. (2016). How many credits should an undergraduate take? Research in Higher Education, 57(6), 682-713. https://doi.org/10.1007/s11162-015-9401-z
Bahr, P. R. (2007). Double jeopardy: Testing the effects of multiple basic skill deficiencies on successful remediation. Research in Higher Education, 48(6), 695-725. https://doi.org/10.1007/s11162-006-9047-y
Bahr, P. R., Jackson, G., McNaughtan, J., Oster, M., & Gross, J. (2017). Unrealized potential: Community college pathways to STEM baccalaureate degrees. The Journal of Higher Education, 88(3), 430-478. https://doi.org/10.1080/00221546.2016.1257313
Bailey, T., Jeong, D. W., & Cho, S. W. (2010). Referral, enrollment, and completion in developmental education sequences in community colleges. Economics of Education Review, 29(2), 255-270. https://doi.org/10.1016/j.econedurev.2009.09.002
Bailey, T. R., Jaggars, S. S., & Jenkins, D. (2015). Redesigning America's community colleges: A clearer path to student success . Harvard University Press.
Belfield, C., Jenkins, D., & Fink, J. (2019). Early momentum metrics: Leading indicators for community college improvement . Columbia University, Teachers College, Community College Research Center. https://ccrc.tc.columbia.edu/publications/early-momentum-metrics-leading-indicators.html
Bickerstaff, S. E., Chavarín, O., & Raufman, J. (2018). Mathematics pathways to completion: Setting the conditions for statewide reform in higher education. Columbia University, Teachers College, Community College Research Center. https://academiccommons.columbia.edu/doi/10.7916/d8-qqt8-3p61
Boatman, A., & Long, B. T. (2018). Does remediation work for all students? How the effects of postsecondary remedial and developmental courses vary by level of academic preparation. Educational Evaluation and Policy Analysis, 40(1), 29-58. https://doi.org/10.3102%2F0162373717715708
Bowen, W. G., Chingos, M. M., & McPherson, M. S. (2018). Test scores and high school grades as predictors. In Buckley, J., Letukas, L., & Wildavsky, B. (Eds.), Measuring success: Testing, grades, and the future of college admissions . Johns Hopkins University Press.
Bryk, A. S., & Treisman, U. (2010, April 18). Make math a gateway, not a gatekeeper. The Chronicle of Higher Education. https://www.chronicle.com/article/Make-Math-a-Gateway-Not-a/65056
Burdman, P., Booth, K., Thorn, C., Bahr, P. R., McNaughtan, J., & Jackson, G. (2018). Multiple paths forward: Diversifying mathematics as a strategy for college success . WestEd & Just Equations. https://www.wested.org/resources/multiple-paths-forward/
Cafarella, B. (2021). Community college faculty perspectives regarding Quantway. Community College Journal of Research and Practice, 45(6), 437-450. https://doi.org/10.1080/10668926.2020.1719940
Charles A. Dana Center. (2016). The case for mathematics pathways. https://dcmathpathways.org/resources/case-mathematics-pathways
Charles A. Dana Center. (2015). Back-to-back math: Strategies for ensuring successive semester enrollment. https://dcmathpathways.org/sites/default/files/2016-08/Back-to-Back%20Math%20Strategies_0.pdf
Cohen, A. M., Brawer, F. B., & Kisker, C. B. (2013). The American community college (6th ed.). Jossey-Bass.
Cohen, J., & Cohen, P. (1983). Applied multiple regression/correlational analysis for the behavioral sciences (2nd ed.). Lawrence Erlbaum Associates.
Finster, M., & Feldman, J. (2021). Cost-effectiveness of a statistics-based approach to developmental mathematics education. Journal of College Student Retention: Research, Theory & Practice . Advance online publication. http://dx.doi.org/10.1177/1521025121996282
Florida College System. (2018). The fact book 2018. Florida Department of Education. http://www.fldoe.org/accountability/data-sys/CCTCMIS/reports.stml
Florida Student Success Center. (2019). Mathematics re-design: A vision for Florida's future. https://www.floridacollegesystemfoundation.org/fssc-math-redesign
Hacker, A. (2016). The math myth and other STEM delusions. The New Press.
Handel, M. (2016). What do people do at work? A profile of U.S. jobs from the survey of workplace Skills, Technology, and Management Practices (STAMP). Journal for Labour Market Research, 49(2), 177-197. http://dx.doi.org/10.1007/s12651-016-0213-1
Harper, S. R., Patton, L. D., & Wooden, O. S. (2009). Access and equity for African American students in higher education: A critical race historical analysis of policy efforts. The Journal of Higher Education, 80(4), 389-414. https://doi.org/10.1080/00221546.2009.11779022
Hern, K. (2010). Exponential attrition and the promise of acceleration in developmental English and math . In Perspectives: Research and Planning Group. https://rpgroup.org/Portals/0/Documents/Projects/California%20Acceleration%20Project%20(CAP)%20Evaluation/Hern%20Exponential%20Attrition.pdf
Hoang, H., Huang, M., Sulcer, B., & Yesilyurt, S. (2017). Carnegie Math Pathways 2015-2016 impact report: A five-year review. Carnegie Foundation for the Advancement of Teaching. https://www.carnegiefoundation.org/resources/publications/carnegie-math-pathways-2015-2016-impact-report-a-five-year-review/
Hoffman, J. L., & Lowitzki, K. E. (2005). Predicting college success with high school grades and test scores: Limitations for minority students. Review of Higher Education, 28(4), 455-474. https://doi.org/10.1353/rhe.2005.0042
Holzer, H. J., & Xu, Z. (2019). Community college pathways for disadvantaged students. Center for Analysis of Longitudinal Data in Education Research (CALDER). https://papers.ssrn.com/sol3/papers.cfm?abstract_id=3390308
Hu, S., Bertrand Jones, T., Brower, R., Nix, A., Martindale, S., Rahming, S., & Tandberg, D. (2016). Learning to adapt: Lessons from the second year of developmental education reform at Florida College Systems institutions . Center for Postsecondary Success. https://doi.org/10.33009/fsu.1485302202
Huang, M. (2018). 2016-2017 Impact report: Six years of results from the Carnegie math pathways . Carnegie Foundation for the Advancement of Teaching. https://www.carnegiefoundation.org/resources/publications/2016-2017-impact-report-six-years-of-results-from-the-carnegie-math-pathways/
Huang, M., & Yamada, H. (2017). Maintaining success rates: Does Statway® sustain its impact as it scales to new classrooms and institutions? Carnegie Foundation for the Advancement of Teaching. https://files.eric.ed.gov/fulltext/ED582441.pdf
Jenkins, D., & Bailey, T. (2017). Early momentum metrics: Why they matter for college improvement. CCRC Brief No. 65. Columbia University, Teachers College, Community College Research Center. https://ccrc.tc.columbia.edu/media/k2/attachments/early-momentum-metrics-college-improvement.pdf
Jenkins, D., Lahr, H., & Fink, J. (2017). Building blocks: Laying the groundwork for guided pathways reform in Ohio . Columbia University, Teachers College, Community College Research Center. https://ccrc.tc.columbia.edu/media/k2/attachments/building-blocks-laying-groundwork-guided-pathways-reform-ohio.pdf
Leeds, D., & Mokher, C. G. (2020). Improving indicators of college readiness: Methods for optimally placing students into multiple levels of postsecondary coursework. Educational Evaluation and Policy Analysis, 42(1), 87-109. https://doi.org/10.3102%2F0162373719885648
Leite, W. L., Aydin, B., & Gurel, S. (2019). A comparison of propensity score weighting methods for evaluating the effects of programs with multiple versions. The Journal of Experimental Education, 87(1), 75-88. https://doi.org/10.1080/00220973.2017.1409179
Mejia, M. C., Rodriguez, O., & Johnson, H. (2019). What happens when colleges broaden access to transfer-level courses. Public Policy Institute of California. https://www.ppic.org/wp-content/uploads/what-happens-when-colleges-broaden-access-to-transfer-level-courses-evidence-from-californias-community-colleges.pdf
Melguizo, T., Hagedorn, L. S., & Cypers, S. (2008). Remedial/developmental education and the cost of community college transfer: A Los Angeles County sample. Review of Higher Education, 31(4), 401-431. https://doi.org/10.1353/rhe.0.0008
Monaghan, D. B., & Attewell, P. (2015). The community college route to the bachelor's degree. Educational Evaluation and Policy Analysis, 37(1), 70-91. https://doi.org/10.3102%2F0162373714521865
Mueller, J. A., & Broido, E. M. (2012). Historical context: Who we were is part of who we are. In J. Arminto, V. Torres, & R.L. Pope (Eds.), Why aren't we there yet? Taking personal responsibility for creating an inclusive campus (pp. 57-103). Stylus.
Ngo, F. J., & Velasquez, D. (2020). Inside the math trap: Chronic math tracking from high school to community college. Urban Education. Advance online publication. https://doi.org/10.1177/0042085920908912
Norman, J. (2017). Pathways post-participation outcomes: Preliminary findings. Carnegie Foundation for the Advancement of Teaching. https://www.carnegiefoundation.org/wp-content/uploads/2017/02/Carnegie_Pathways_PPP_Mar2017.pdf
Park, T. J., Tandberg, D. A., Hu, S., & Hankerson, D. (2016). One policy, disparate reactions: Institutional responses in Florida's developmental education reform. Community College Journal of Research and Practice, 40(10), 824-837. https://doi.org/10.1080/10668926.2015.1131644
Provasnik, S., & Planty, M. (2008). Community colleges: Special supplement to the condition of education 2008 (NCES 2008-033). U.S. Department of Education, Institute of Education Sciences, National Center for Education Statistics. https://nces.ed.gov/pubs2008/2008033.pdf
Purnell, R. D., & Burdman, P. (2021). Go figure: Exploring equity in students' postsecondary math pathway choices. Journal of Higher Education Theory & Practice, 21(11), 155-185. https://doi.org/10.33423/jhetp.v21i11.4673
Reynolds, C. L., & DesJardins, S. L. (2010). The use of matching methods in higher education research. In J. C. Smart (Ed.), Higher education: Handbook of theory and research (Vol. 24; pp. 47-104). Springer.
Rutschow, E. Z. (2018). Making it through: Interim findings on developmental students' progress to college match with the Dana Center Mathematics Pathways . Columbia University, Teachers College, Center for the Analysis of Postsecondary Readiness. https://postsecondaryreadiness.org/interim-findings-dana-center-mathematics-pathways/
Rutschow, E. Z., & Diamond, J. (2015). Laying the foundations: Early findings from the New Mathways Project . MDRC. https://www.mdrc.org/sites/default/files/New_Mathways_ES.pdf
Rutschow, E. Z., Diamond, J., & Serna-Wallender, E. (2017). Math in the real world: Early findings from a study of the Dana Center Mathematics Pathways . Columbia University, Teachers College, Center for the Analysis of Postsecondary Readiness. https://www.mdrc.org/sites/default/files/2017_MathRealWorld.pdf
Rutschow, E. Z., & Mayer, A. K. (2018). Early findings from a national survey of developmental education practices. Research brief . Center for the Analysis of Postsecondary Readiness. https://eric.ed.gov/?id=ED583573
Schudde, L., & Keisler, K. (2019). The relationship between accelerated dev-ed coursework and early college milestones: Examining college momentum in a reformed mathematics pathway. AERA Open, 5(1). https://doi.org/10.1177%2F2332858419829435
Schudde, L., & Meiselman, Y. (2019). Assessing the influence of Dana Center Mathematics Pathways on dev-ed math students' early college outcomes: Fall 2015 and 2016 cohorts . National Academy of Sciences. http://sites.nationalacademies.org/cs/groups/dbassesite/documents/webpage/dbasse_191809.pdf
Scott-Clayton, J. (2011). The shapeless river: Does a lack of structure inhibit students' progress at community colleges? CCRC Working Paper No. 25. Columbia University, Teachers College, Community College Research Center. https://files.eric.ed.gov/fulltext/ED515131.pdf
Scott-Clayton, J., Crosta, P. M., & Belfield, C. R. (2014). Improving the targeting of treatment: Evidence from college remediation. Educational Evaluation and Policy Analysis, 36(3), 371-393. https://doi.org/10.3102%2F0162373713517935
Underhill, B. (2013). College remediation. Presentation at the Florida Senate the 2013 Regular Session Appropriations Subcommittee on Education Meeting. http://www.flsenate.gov/PublishedContent/Committees/2012-2014/AED/MeetingRecords/MeetingPacket_2056.pdf
Wang, X. (2016). Course-taking patterns of community college students beginning in STEM: Using data mining techniques to reveal viable STEM transfer pathways. Research in Higher Education, 57(5), 544-569. https://doi.org/10.1007/s11162-015-9397-4
What Works Clearinghouse. (2020). What Works Clearinghouse: Standards handbook (Version 4.1). Institute of Education Sciences, U.S. Department of Education. https://ies.ed.gov/ncee/wwc/Docs/referenceresources/WWC-Standards-Handbook-v4-1-508.pdf
Yamada, H., Bohannon, A. X., Grunow, A., & Thorn, C. A. (2018). Assessing the effectiveness of Quantway®: A multilevel model with propensity score matching. Community College Review, 46(3), 257-287. https://doi.org/10.1177%2F0091552118771754
*Contact: cmokher@fsu.eduJournal of Postsecondary Student Success, Volume 1, Issue 3